Artificial intelligence is transforming businesses—and predictive AI is one of the most valuable applications for driving growth and efficiency. In this post, we'll explore how predictive AI works and the key ways it can impact organizations.
What is Predictive AI?
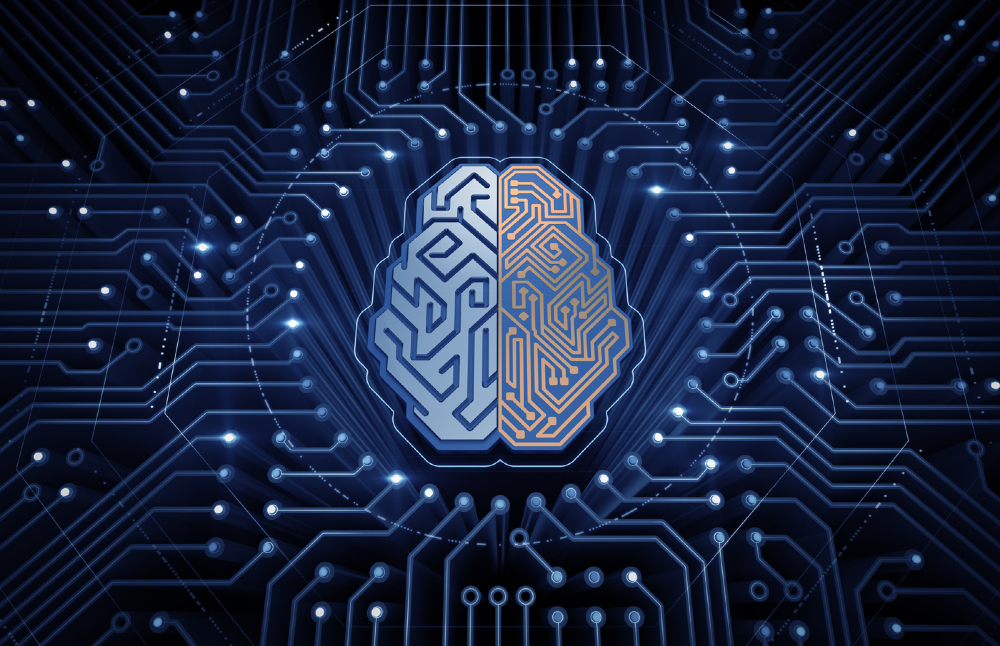
Predictive AI is a technique that analyzes current and historical data to identify patterns and anticipate potential outcomes. The core goal is to forecast trends, risks, and opportunities.
Predictive AI leverages the same principle as big data analytics—larger data volumes enable more sophisticated predictive modeling, and machine learning algorithms identify correlations in the data to make predictions representing different scenarios.
Unlike descriptive analytics (which reports on what happened) or prescriptive analytics (which recommends actions), predictive AI focuses solely on predicting the future based on data insights.
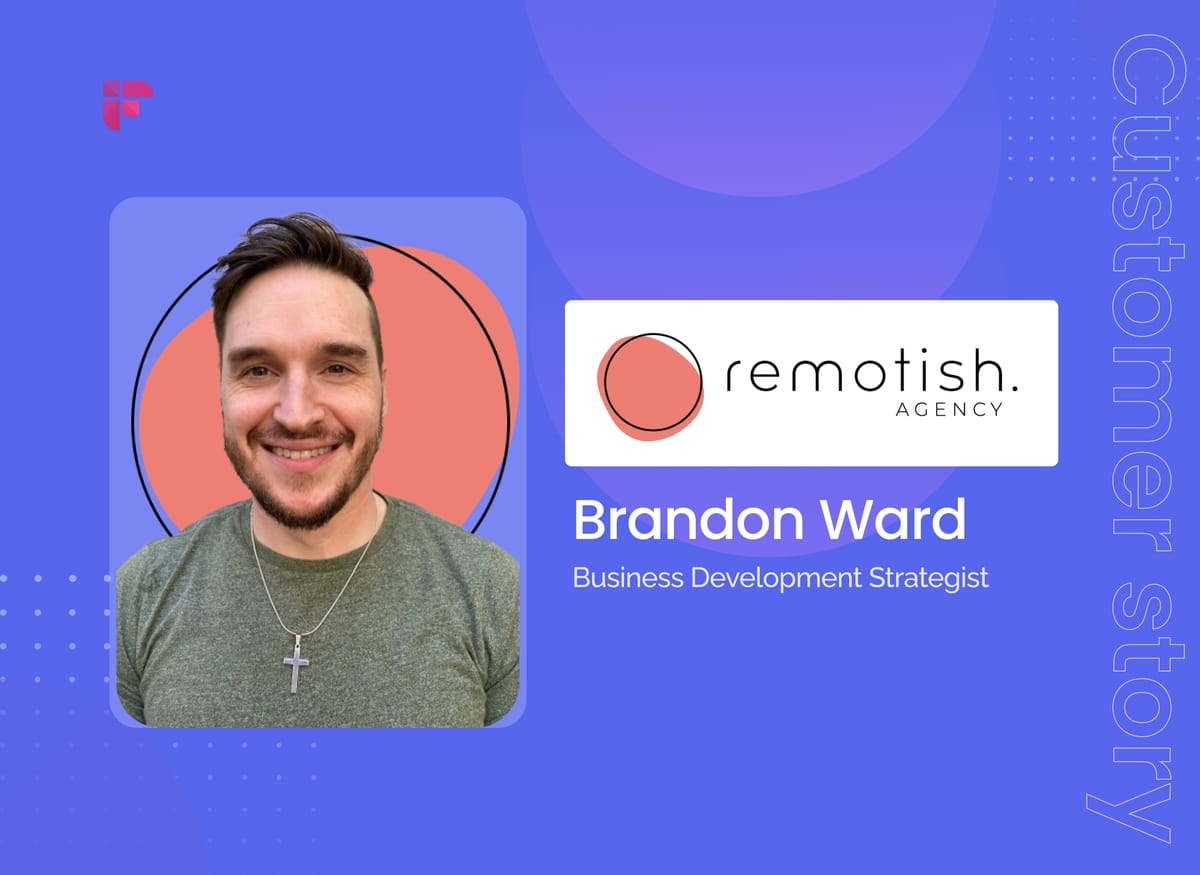
How Does Predictive AI Work?
There are a few key capabilities that enable predictive AI to forecast future outcomes:
Data analysis
Predictive AI ingests large volumes of historical data relevant to the problem. First, This data is aggregated from different sources across the organization. Machine learning algorithms then analyze this data to identify trends, patterns, and relationships between variables.
Statistical modeling
Various statistical and machine learning techniques are used to train predictive models on the prepared historical datasets. Common modeling methods include regression analysis, decision trees, neural networks, time series forecasting, and ensemble modeling.
Model evaluation
The trained models are rigorously tested using test data sets to evaluate their accuracy and precision. The models are refined until the desired level of predictive performance is achieved.
Scenario simulation
With reasonably accurate models, data scientists can simulate different scenarios by tweaking input parameters to estimate forecasts under various conditions.
Model deployment
Once satisfactory models are developed, they are deployed into production environments. New data can be continuously fed into the models to generate updated predictive insights.
Process integration
Finally, the predictive insights are integrated into business processes and workflows through dashboards, alerts, APIs, etc., to enable data-driven decision-making based on the model forecasts.
The availability of rich data combined with computing power and advanced algorithms makes predictive AI so powerful and valuable for organizations today. The models become more intelligent over time as they process more information.
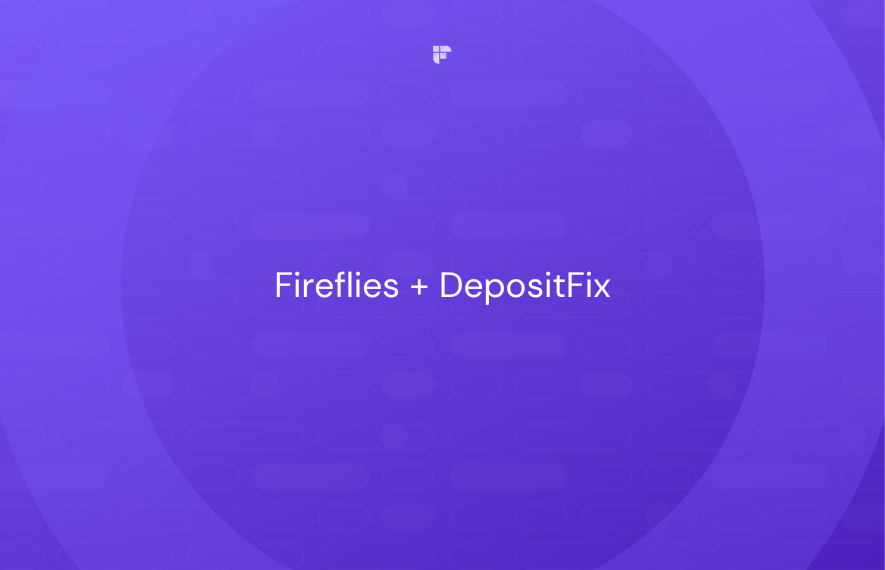
Predictive AI use cases
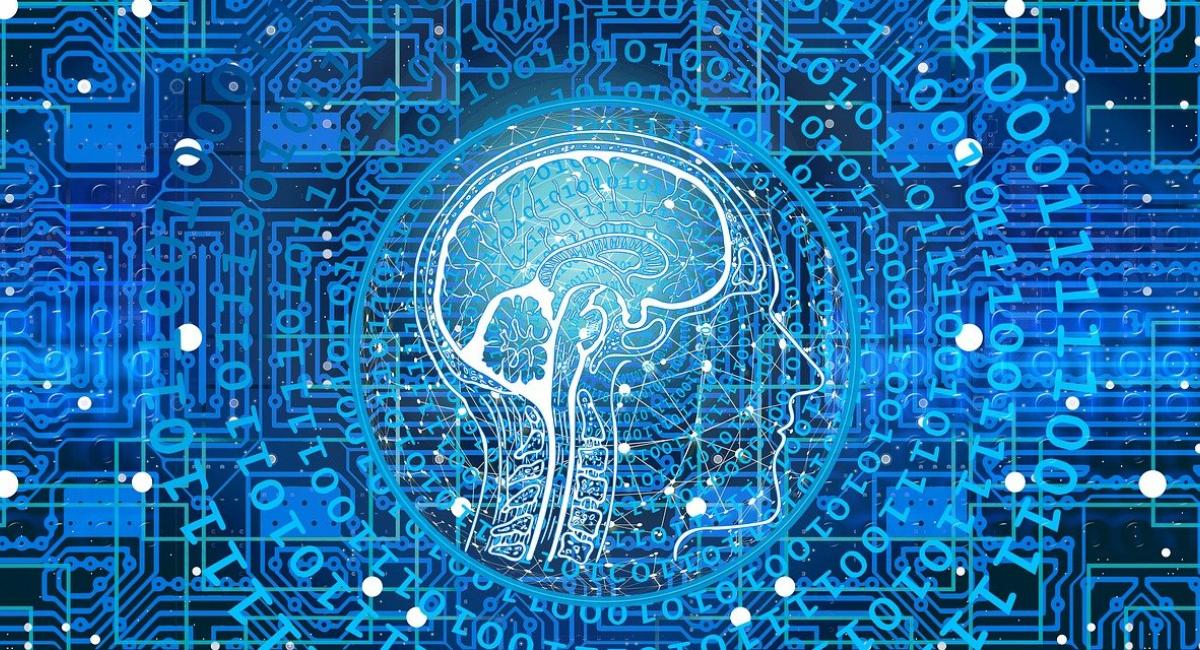
Predictive analytics and AI enable organizations across all major industries to optimize operations, reduce costs and risks, and identify new opportunities.
- Customer retention
- Dynamic pricing
- Predictive maintenance
- Supply chain optimization
- Healthcare Analytics
Customer retention
- Analyze customer data to build churn prediction models that identify high-risk customers.
- Proactively engage at-risk customers with tailored incentives and communication channels to influence them to stay.
- Significantly improve customer retention rates and lifetime value.
Dynamic pricing
- Ingest data on demand, competitors, seasons, events, and other external factors to frequently adjust pricing.
- Price optimization algorithms maximize revenue and profitability by adapting to real-time changes.
- Maintain a competitive edge with data-driven personalized pricing.
Predictive maintenance
- Monitor IoT sensor data from industrial equipment and infrastructure to detect anomalies and predict maintenance needs.
- Schedule proactive maintenance to prevent unplanned downtime and catastrophic failures before they occur.
- Optimize maintenance costs by aligning to actual requirements forecasted by AI models.
Supply chain optimization
- Predict expected demand across regions, stores, and online channels to optimize inventory levels.
- AI-enabled demand forecasting minimizes waste, shortage, and inventory carrying costs.
- Dynamic inventory allocation and distribution maximize service levels.
Healthcare Analytics
- Identify patients at risk of readmission or disease based on clinical and lifestyle data.
- Enable precision medicine and preventive care optimized to an individual's health needs predicted by AI.
- Improve patient outcomes and lower healthcare costs.
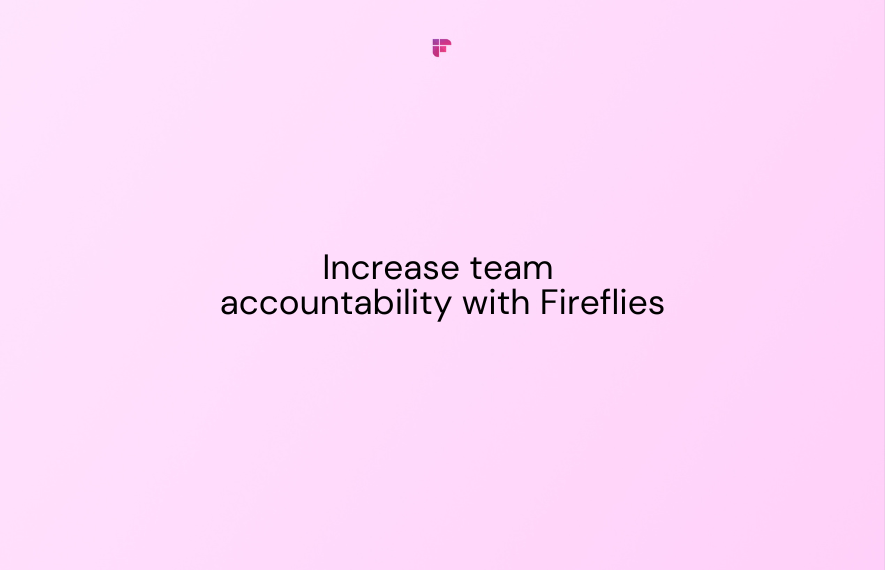
Predictive AI vs. Generative AI
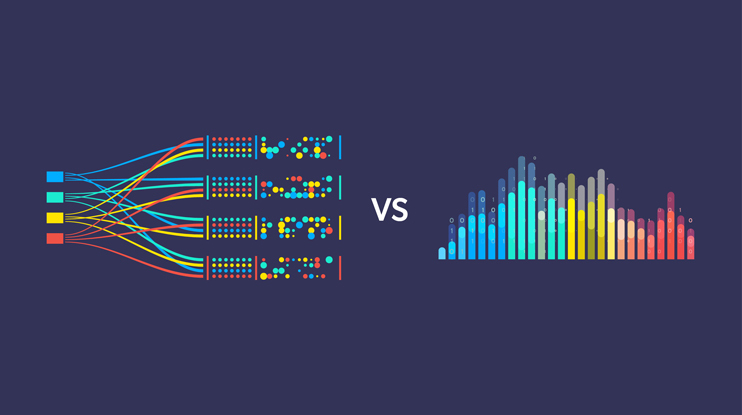
Predictive AI and Generative AI represent two emerging branches of artificial intelligence that are often conflated. However, there are some key differences:
- Predictive AI analyzes data to forecast potential future outcomes. Generative AI creates entirely new data or content.
- Predictive models extrapolate from existing datasets. Generative models create original artifacts like images, video, text, etc. Here are 10 Generative AI use cases that will change how you work
- Predictive AI aims to anticipate future events and behaviors. Generative AI aims to mimic human creativity.
- Common predictive techniques involve regression and neural networks. Generative models leverage GANs, VAEs, and reinforcement learning.
- Predictive analytics identifies trends. Generative algorithms generate simulated samples.
Leverage Generative AI in your meetings.
Use Fireflies.ai to transcribe, summarize, analyze your meetings, and create follow-up content using its AI assistant, AskFred.
Try Fireflies for free!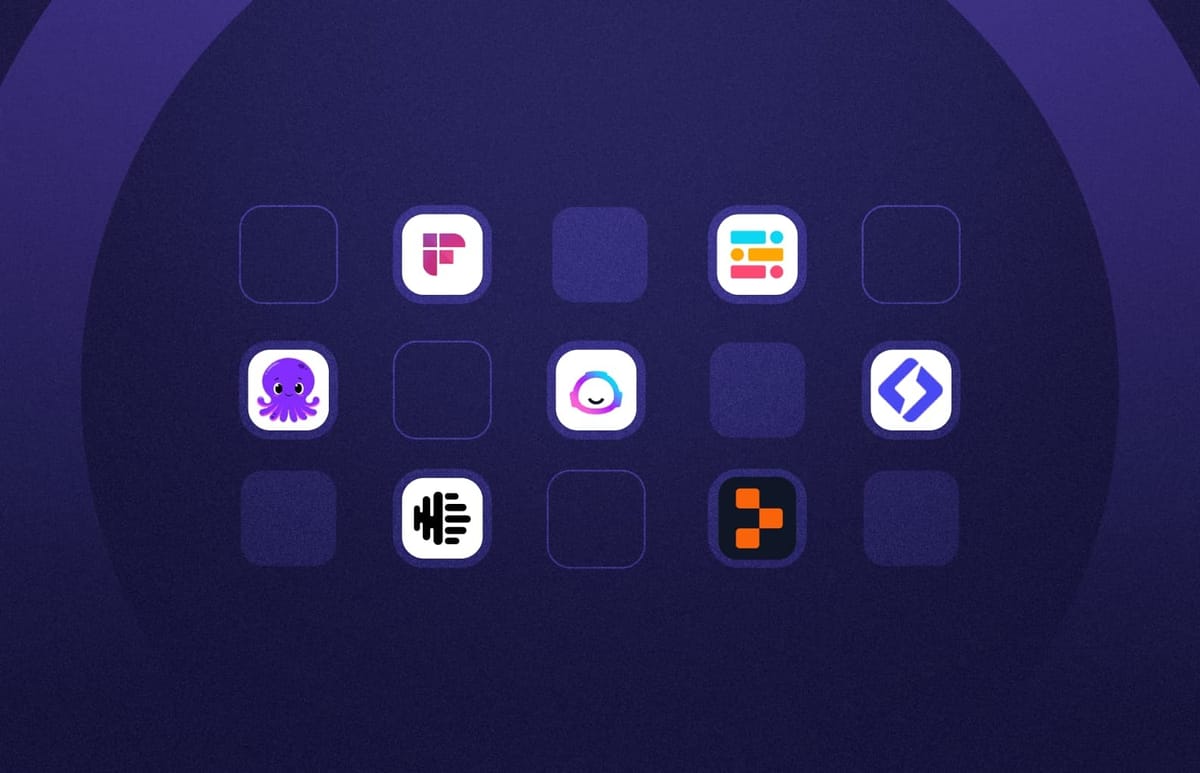
The future is predictive
The potential of predictive AI as a driver for business growth and efficiency cannot be overstated. Its ability to turn vast amounts of data into actionable insights presents a game-changing opportunity for organizations across industries.
As we continue to refine these technologies and integrate them more deeply into our operational processes, the future of business looks not just predictable but bright.