Predictive AI and generative AI stand out in the AI landscape, each with unique strengths and versatile applications.
One predicts the future, while the other generates fresh, innovative content. But beyond just being buzzwords, what's the real deal behind them?
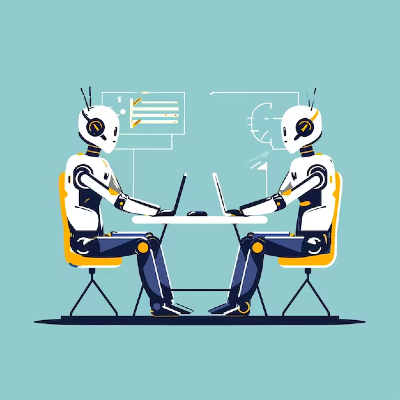
Read this Predictive AI vs. Generative AI article to find out.
Also read: AI Assistants: Everything You Need to Know
What is predictive AI?
Predictive AI is a branch of artificial intelligence that uses various statistical methods and machine learning algorithms to identify patterns in historical data and make predictions about future events.
These predictions can range from short-term forecasts (e.g., predicting customer churn within the next month) to long-term trends (e.g., predicting market fluctuations years ahead).
For example, retail giant Walmart predicts its inventory needs months ahead by running analytical models on years of sales data revealing seasonal and cyclical patterns. Similarly, neuroscientists feed scans showing biomarkers into machine learning systems that estimate Alzheimer's onset long before symptoms arise.
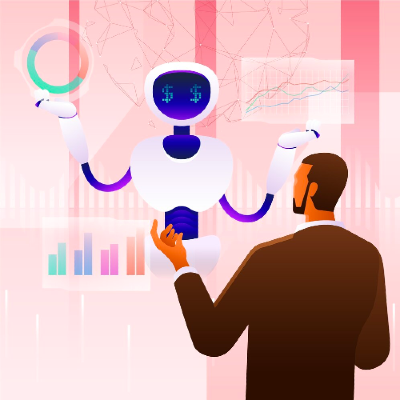
Let’s take a look at some specific applications of predictive AI:
- Maintenance: Predict equipment failures by analyzing IoT data for timely maintenance.
- Fraud detection: Detect fraud in real-time by identifying suspicious transactional patterns.
- Cybersecurity: Defend against cyberattacks by recognizing new malicious activity.
- Healthcare: Personalize healthcare via data-driven treatment, recommendations, and early diagnosis.
- Finance advisory: Enable algorithmic trading by processing signals and trends in stock markets.
- Marketing: Boost marketing ROI through predictive lead scoring and dynamic campaign optimization.
What techniques power predictive analytics?
- Regression analysis: Assesses how independent variables impact specific outcomes.
- Time series analysis: Reveals seasonal/cyclic patterns useful for forecasting.
- Random forests: Ensemble machine learning method combining decision tree predictors.
- Neural networks: Mimics human neuron interconnections to uncover hidden correlations.
Learn more about predictive AI.
Current limitations of Predictive AI
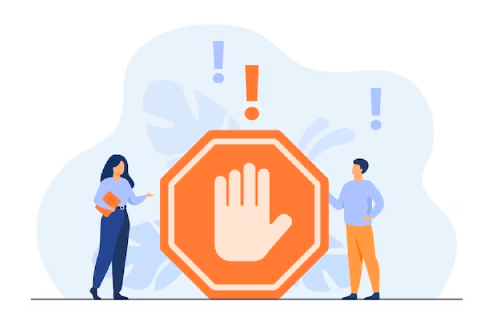
While promising, predictive AI does have some limitations:
- Result reliability depends heavily on training data quality. Insufficient or biased data causes skewed projections.
- Understanding how predictive AI models arrive at their predictions can be difficult, especially for complex models like artificial neural networks. This opacity poses a challenge, especially when regulations demand model transparency. In such cases, simpler models that meet regulatory standards are preferred.
- Rare, unexpected events, commonly known as black swans, remain difficult to integrate into forecasts. Even the most sophisticated models cannot account for unpredictable geopolitical or environmental impacts.
- Continuous model retraining is imperative as markets and external and internal dynamics evolve. Teams need resources for constant incremental enhancement.
What is generative AI?
Unlike predictive models anticipating likely futures, generative AI focuses on creating new and original content.
Generative AI trains on large, diverse datasets like text, images, or speeches to understand creative styles, themes, and relationships. It then renders the original output adhering to patterns in the training data.
The objective is to mimic human creativity. For instance, text or image inputs are used to compose music, generate poems, graphic design, visual 3D models, or videos.
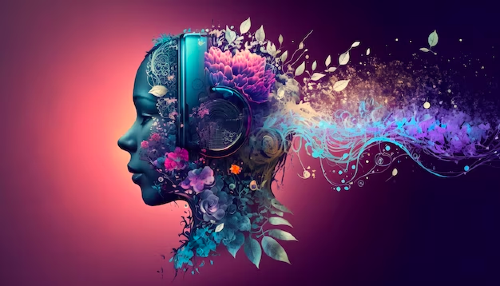
Leading examples of generative AI include systems like DALL-E 2, ChatGPT, Google Gemini (prev. Bard), and Anthropic’s Claude.
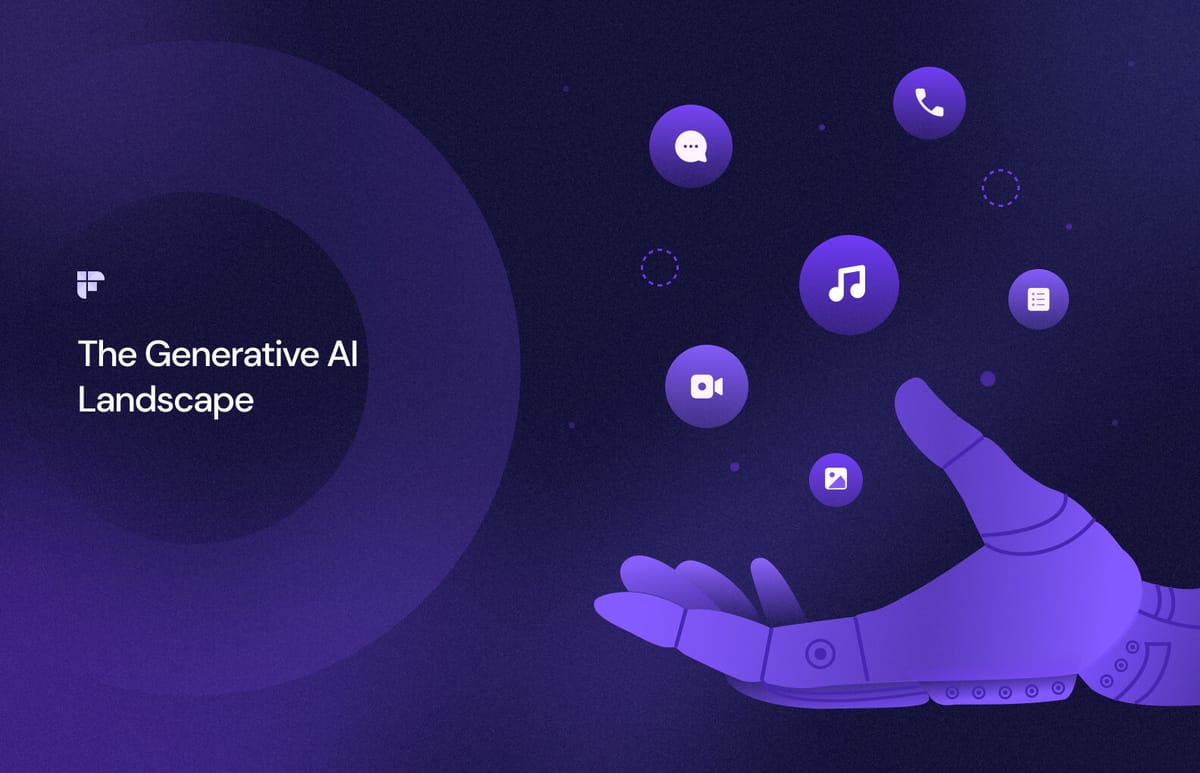
Typical use cases of generative AI:
- Marketing: Generate personalized campaigns, dynamic product descriptions, blogs, social media posts, and tailored ad creatives to boost marketing ROI.
- Sales: Generate customized sales presentations, data-driven pitches, and personalized emails and messages for prospects.
- Healthcare: Create patient education materials tailored to profiles, accelerate research paper drafting, and synthesize patient data to train diagnostic AI.
- Finance: Automate client portfolio commentary and reporting, customize policy documents, and simulate trading environments for testing.
- Engineering: Rapidly iterate 3D models and prototypes, generate custom part and product design prompts, and create interactive documentation.
- Education: Personalize lessons, assignments, lectures, and activities for students.
- Media and Entertainment: Procedurally build 3D worlds for games, automate video/music/art generation, and build data-driven character and story development.
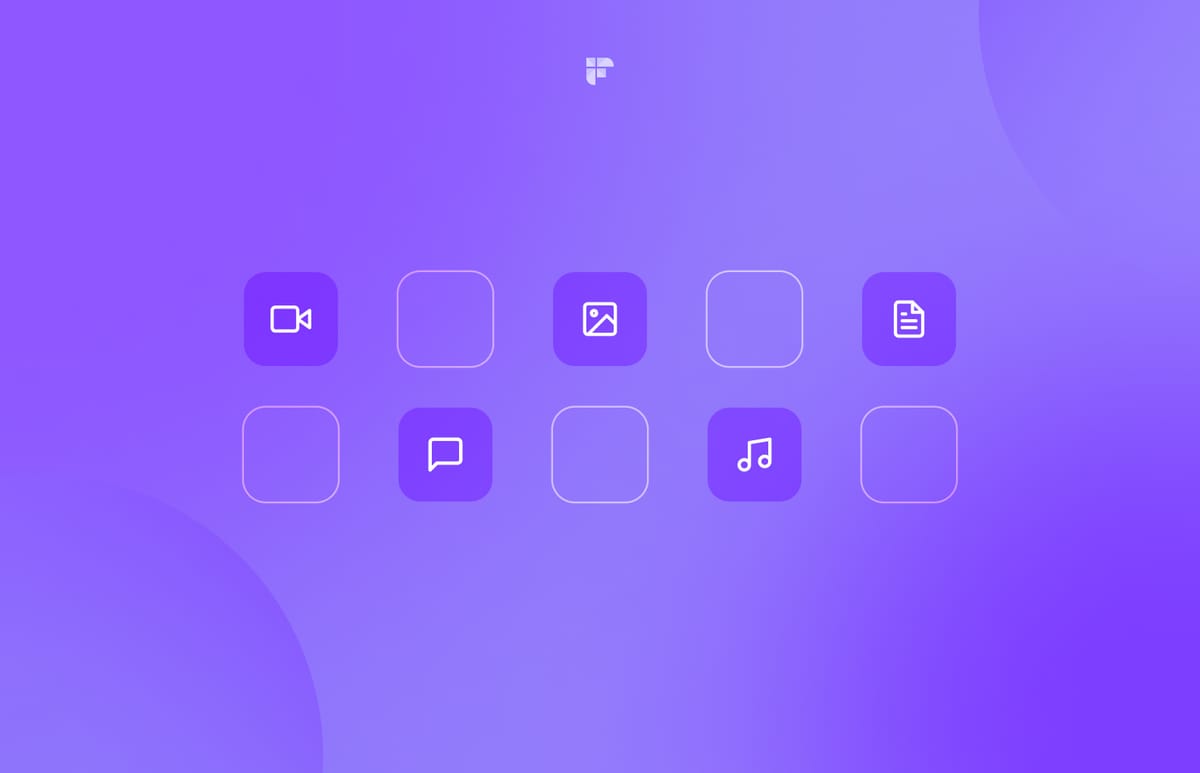
Current limitations of generative AI
Here are some limitations of generative AI:
- Risk of perpetuating and amplifying societal biases. Models trained on biased data propagate problematic patterns in outputs.
- Incoherent or logically unsound content. Generated text or media can include factual gaps or lack overall coherence.
- High computing requirements during training. State-of-the-art generative models can have massive architectures with hundreds of billions of parameters. Training them emits substantial carbon, necessitating environmental responsibility.
- Generative models often lack true creativity and struggle with tasks requiring a genuine understanding of the world. They excel at analyzing existing patterns but struggle to grasp nuance and context.
- Deepfakes and other manipulated media generated by AI pose significant risks in terms of misinformation, propaganda, and social manipulation.
💡 Explore the power of generative AI for meetings with Fireflies.ai!
Get accurate transcripts and meeting summaries. Use the AI-powered chatbot AskFred to ask questions, extract meeting insights, and generate content like blogs, emails, social media posts, and more.
Predictive AI vs. Generative AI
Predictive AI and generative AI leverage machine learning in very different ways to achieve unique outcomes.
Predictive AI analyzes historical data to uncover patterns and insights that inform reasonable predictions about future events. It serves an informational role, amplifying strategic foresight for decision-makers across industries.
Generative AI focuses on producing original content like text, images, video, and music. It trains on diverse datasets to understand creative styles, themes, and relationships within a domain. Generative AI then renders new outputs adhering to patterns in the training data.
Here’s a predictive AI vs. generative AI overview:
While their objectives seem disparate, predictive and generative AI can play complementary roles in building smarter, more assistive AI systems.
Final thoughts
And there you have it, folks—Predictive AI vs. generative AI showdown. Predictive AI offers strategic foresight, while Generative AI sparks creativity.
Each has limitations—predictive AI can lack accuracy without sufficient high-quality data, while generative AI risks perpetuating societal biases and lacking coherence.
While their objectives differ and both have limitations, they play complementary roles in building more innovative, assistive AI systems. Together, they demonstrate AI's versatile potential to anticipate future or imagine new realities.